The first wave of the SARS-CoV-2 epidemic in Tuscany (Italy): a SI²R²D compartmental model with uncertainty evaluation
Publication date: 29/10/2020 – E&P Code: repo.epiprev.it/2003
Authors: Michela Baccini1, Giulia Cereda1, Cecilia Viscardi1
Abstract: With the aim of studying the spread of the SARS-CoV-2 infection in the Tuscany region of Italy during the first epidemic wave (from February to June 2020), we define a compartmental model which accounts for both detected and undetected infections and assumes that only notified cases can die. We estimate the initial infection and case fatality rates and the basic reproduction number, modeled as a time-varying function, by calibrating on the cumulative daily number of observed deaths and notified infected, after fixing to plausible values the other model parameters to assure identifiability. The uncertainty of the estimates is quantified by a parametric bootstrap procedure and a Global Sensitivity Analysis (GSA) based on the Sobol’s variance decomposition is performed to assess the sensitivity of the estimates to changes in the values of the fixed parameters. According to our results, the basic reproduction number drops from an initial value of 6.055 to 0 at the end of the national lockdown, then it grows again, but remaining under 1. At the beginning of the epidemic, the case and the infection fatality rates are estimated to be 13.1% and 2.3%, respectively. Among the parameters considered as fixed, the average time from infection to recovery for the not notified infected appears to be the most impacting one on the model estimates. The probability for an infected to be notified has a relevant impact on the infection fatality rate and on the shape of the epidemic curve. This stresses the need of collecting information on these parameters to better understand the phenomenon and get reliable predictions.
Cite as: Michela Baccini, Giulia Cereda, Cecilia Viscardi (2020). The first wave of the SARS-CoV-2 epidemic in Tuscany (Italy): a SI²R²D compartmental model with uncertainty evaluation. E&P Repository https://repo.epiprev.it/2003
Topic: COVID-19
Key words: COVID-19, infection fatality rate, modelli compartimentali, parametric boostrap, SARS-CoV-2,
AVVERTENZA. GLI ARTICOLI PRESENTI NEL REPOSITORY NON SONO SOTTOPOSTI A PEER REVIEW.
Info
Affiliations:
1 Dipartimento di Statistica, Informatica, Applicazioni, Università di Firenze, Florence Center for Data Science, Università di Firenze
Authors’ contributions: –
Competing interests: none.
Funding disclosure: –
Ethics committee approval: The study was based on publicly available aggregate data. No Ethics committee approval was necessary.
Copyright: Il detentore del copyright è l’autore/finanziatore, che ha concesso a “E&P Repository” una licenza per rendere pubblico questo preprint. The copyright holder for this preprint is the author/funder, who has granted E&P Repository a license to display the preprint in perpetuity.
Terms of distribution: CC BY-NC-ND
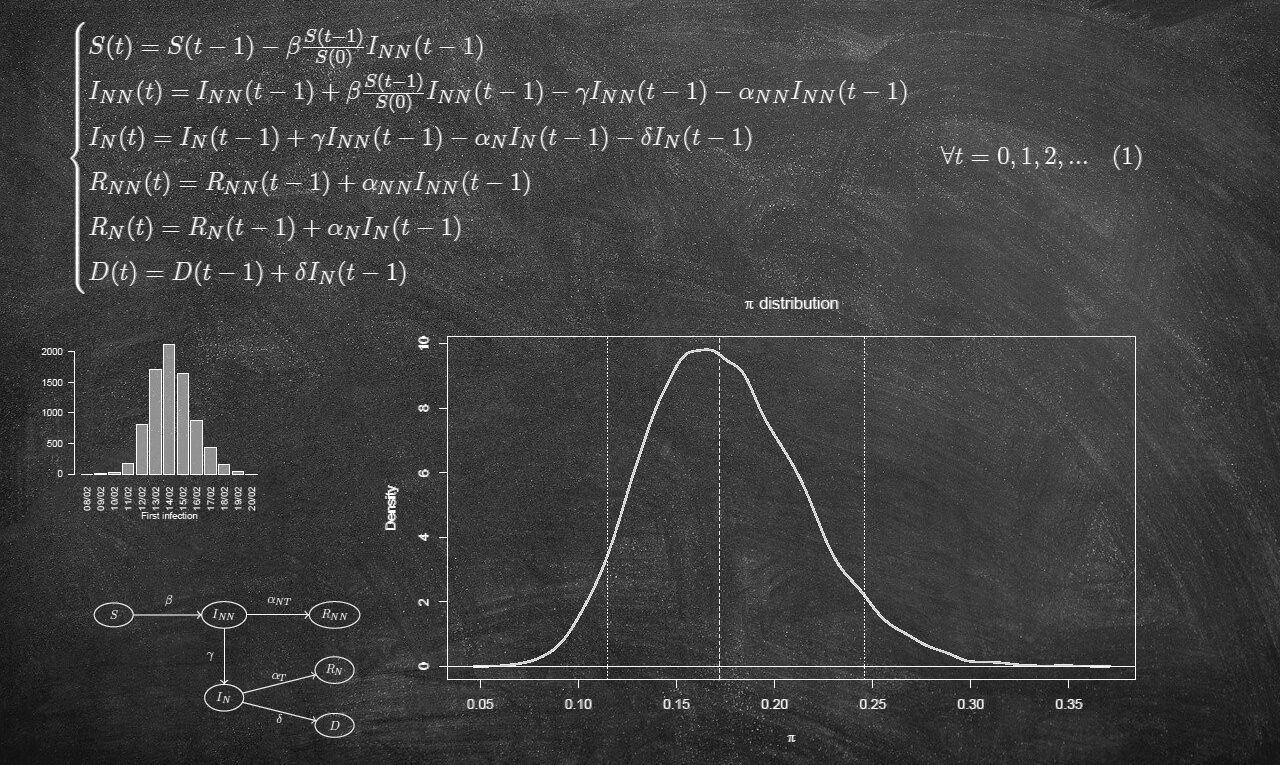
Immagine redazionale con funzione decorativa che riproduce alcuni elementi del pre-print. Fonte: adattamento immagine da pexels.com (1072366)
References & Citations
Google Scholar