A reanalysis of an Italian study on the effectiveness of COVID-19 vaccination suggests that it might have unintended effects on total mortality
Publication date: 11/04/2024 – E&P Code: repo.epiprev.it/2862
Authors: Alessandria M.1, Malatesta G.2, Donzelli A.3, Berrino F.4.
Abstract: Immortal-time bias (ITB) is known to be common in cohort studies and distorts the association estimates between treated and untreated groups. We used data from the last of two large studies in an Italian province on COVID-19 vaccines safety and effectiveness incurred this bias, and aligned the entire population on a single index date, to correct the ITB. We considered the “all-cause deaths” outcome to compare the survival curves between the unvaccinated group and the various vaccination statuses. The all-cause deaths Hazard Ratios in univariate analysis for unvaccinated (reference) versus vaccinated with 1, 2, 3/4 doses were 0.88 (CI95: 0.78 –1.00; p-value 0.044), 1.23 (1.16–1.32; p-value ≤0.001) and 1.21 (1.14–1.29; p-value ≤0.001), respectively. The multivariate values were 2.40 (2.00–2.88; p-value <0.0001), 1.98 (1.75–2.24; p-value <0.0001), 0.99 (0.90–1.09; ns). The possible explanations of the trend of the Hazard Ratios as vaccinations increase could be a harvesting effect; a calendar-time bias, accounting for seasonality and pandemic waves; a case-counting windows bias; a healthy-vaccinee bias; or some their combination. With two and even with 3/4 doses the calculated Restricted Mean Survival Time and Restricted Mean Time Lost have shown a small but significant downside for the vaccinated populations.
Cite as: Alessandria M., Malatesta G., Donzelli A., Berrino F. (2024). A reanalysis of an Italian study on the effectiveness of COVID-19 vaccination suggests that it might have unintended effects on total mortality. E&P Repository https://repo.epiprev.it/2862
Topic: COVID-19
Key words: all-cause deaths, COVID-19 vaccines, Healthy-vaccinee bias, immortal-time bias,
AVVERTENZA. GLI ARTICOLI PRESENTI NEL REPOSITORY NON SONO SOTTOPOSTI A PEER REVIEW.
Info
Affiliations:
1 Department of Life Sciences and Systems Biology, University of Turin, Turin (Italy);
2 Scientific Committee Foundation “Allineare Sanità e Salute”, Pistoia, Italy;
3 Specialist in Hygiene and Preventive Medicine, independent Medical-Scientific Commission; President of Foundation “Allineare Sanità e Salute”, Milan, Italy;
4 Past Director, Department of Predictive and Preventive Medicine, Fondazione IRCCS Istituto Nazionale dei Tumori, Milan, Italy
Authors’ contributions:
Conceptualization A.M., M.G., D.A., B.F.
Data Curation A.M., M.G., D.A., B.F
Formal Analysis A.M., M.G., D.A., B.F
Funding Acquisition D.A.
Investigation A.M., M.G., D.A., B.F
Methodology A.M., M.G., D.A., B.F
Resources A.M., M.G., D.A., B.F
Software A.M
Supervision A.M., M.G., D.A., B.F
Validation A.M., M.G., D.A., B.F
Visualization A.M., M.G., D.A., B.F
Writing – Original Draft Preparation A.M., M.G., D.A., B.F
Writing – Review & Editing A.M., M.G., D.A., B.F
Competing interests: –
Funding disclosure: None
Ethics committee approval: The study was based on publicly available aggregate data. No Ethics committee approval was necessary.
Copyright: Il detentore del copyright è l’autore/finanziatore, che ha concesso a “E&P Repository” una licenza per rendere pubblico questo preprint. The copyright holder for this preprint is the author/funder, who has granted E&P Repository a license to display the preprint in perpetuity.
Terms of distribution: No riutilizzo
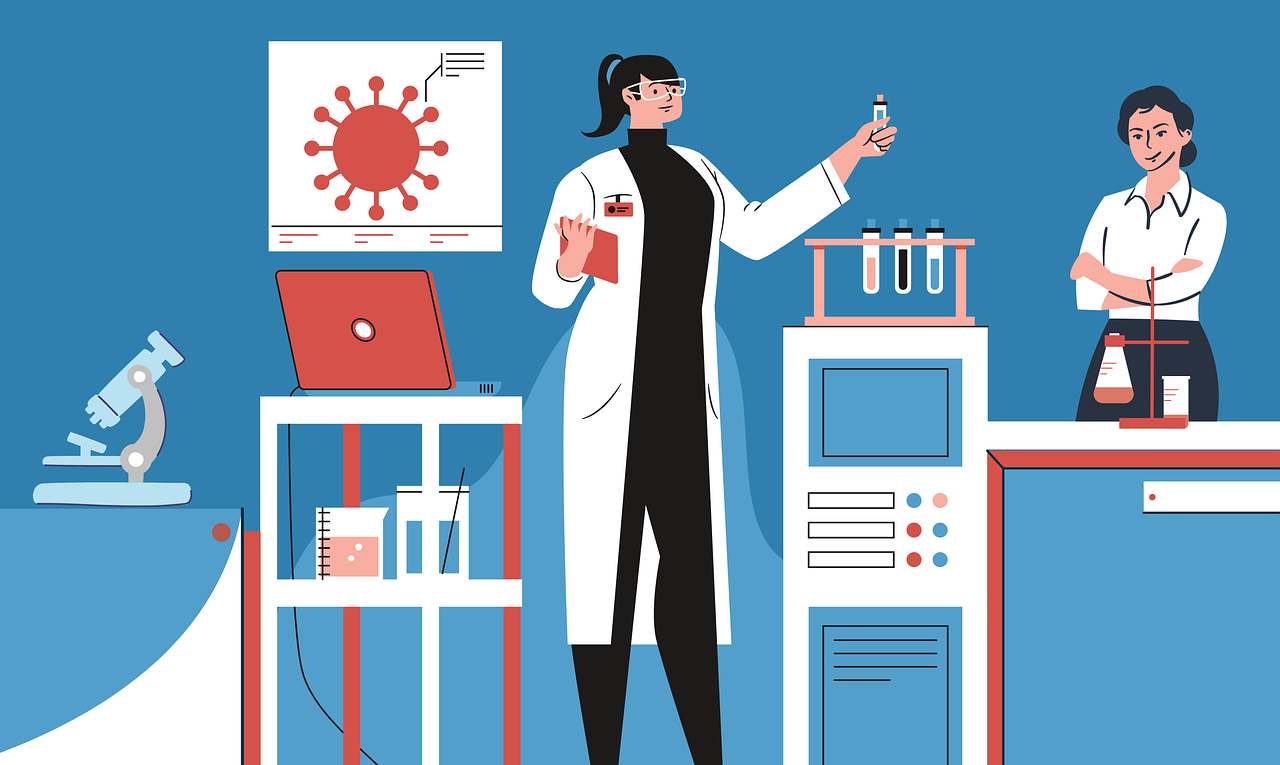
Immagine di redazione. Foto di Moondance da Pixabay
References & Citations
Google Scholar
3 commenti su “A reanalysis of an Italian study on the effectiveness of COVID-19 vaccination suggests that it might have unintended effects on total mortality”